See more publications and researches in Fang-Lue Zhang's homepage.
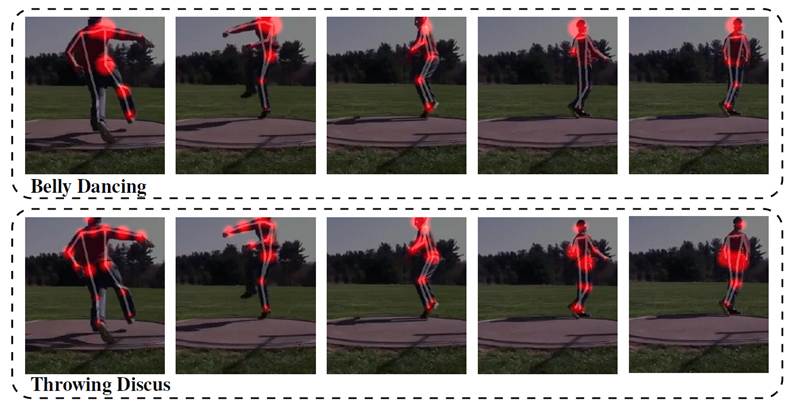
Graph CNNs with Motif and Variable Temporal Block for Skeleton-based Action Recognition
[TPAMI 2022, AAAI 2019, download the paper] Hierarchical structure and different semantic roles of joints in human skeleton convey important information for action recognition. Conventional graph convolution methods for modeling skeleton structure consider only physically connected neighbors of each joint, and the joints of the same type, thus failing to capture high order information. In this work, we propose a novel model with motif-based graph convolution to encode hierarchical spatial structure, and a variable temporal dense block to exploit local temporal information over different ranges of human skeleton sequences. Moreover, we employ a non-local block to capture global dependencies of temporal domain in an attention mechanism. Our model achieves improvements over the state-of-the-art methods on two large-scale datasets.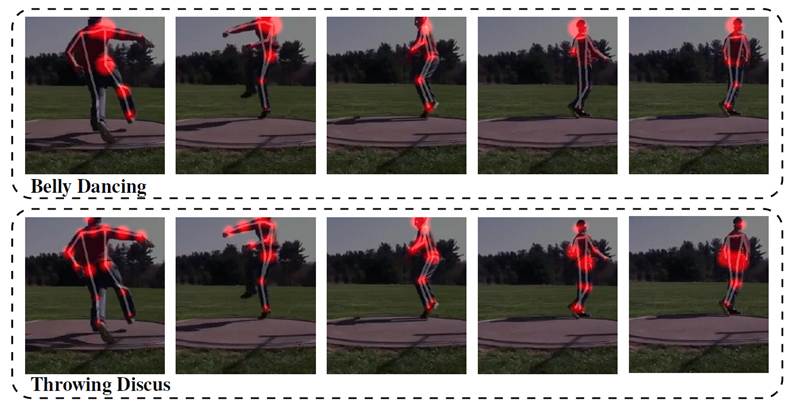
SF-Net: Learning Scene Flow from RGB-D Images with CNNs
With the rapid development of depth sensors, RGB-D data has become much more accessible. Scene flow is one of the fundamental ways to understand the dynamic content in RGB-D image sequences. Traditional approaches estimate scene flow using registration and smoothness or local rigidity priors, which is slow and prone to errors when the priors are not fully satisfied. To address such challenges, learning based methods provide an attractive alternative. In this work, we propose a novel learning-based framework to estimate scene flow, which takes both brightness and scene flow losses. Given a pair of RGB-D images, the brightness loss is used to measure the disparity between the first RGB-D image and the deformed second RGB-D image using the scene flow, and the scene flow loss is used to learn from the ground truth of scene flow. We build a convolutional neural network to simultaneously optimize both losses. Extensive experiments on both synthetic and real-world datasets show that our method is significantly faster than existing methods and outperforms state-of-the-art real-time methods in accuracy. [BMVC 2018, download]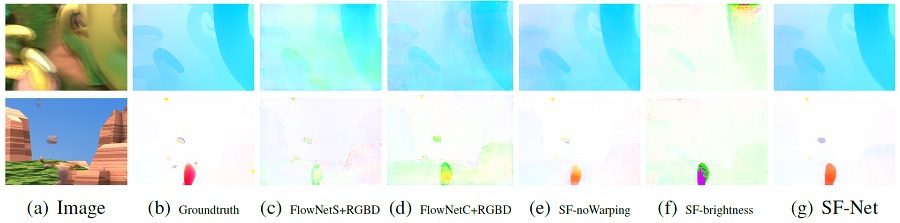
I | Attachment | Action | Size | Date | Who | Comment |
---|---|---|---|---|---|---|
![]() |
Graph CNNs with Motif and Variable Temporal Block for Skeleton-based Action Recognition.pdf | manage | 1 MB | 21 Apr 2019 - 16:56 | Main.fanglue | |
![]() |
Motif.jpg | manage | 50 K | 21 Apr 2019 - 16:49 | Main.fanglue | |
![]() |
SFnet.jpg | manage | 52 K | 21 Apr 2019 - 16:48 | Main.fanglue |