Technology Themes
Theme 1: Data-Driven Methods of Symbolic Learning and Feature Engineering
Leaders:
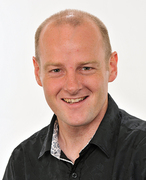
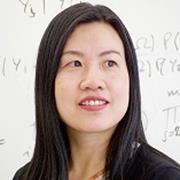
Research Directions:
- Fundamental research to extend causal evolutionary learning methods, initially to improve general performance, but with a longer-term focus on extensions that are more specific to aquaculture-relevant problems
- Fundamental research into symbolic learning and feature selection using ordinal and nominal data
- Fundamental research into regularisation methods for symbolic learning, including multi-objective approaches to regularisation (overlaps with Theme 4, and likely to involve feature construction and selection techniques)
Theme 2: Novel Evolutionary Approaches to Image Recognition and Analysis
Leaders:
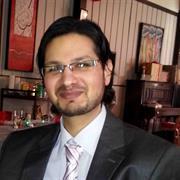
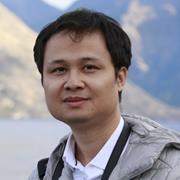
Research Directions:
- Utilise Evolutionary Computation techniques to automatically evolving Deep Learning architectures for image classification/object recognition
- Utilise Evolutionary Computation and Machine Learning techniques for feature extraction (automatically evolving image descriptors and identifying region of interest)
- Utilise Evolutionary Computation and Machine Learning techniques for image enhancement/per-processing and denoising
Theme 3: Transfer Learning and Domain Adaptation
Leaders:
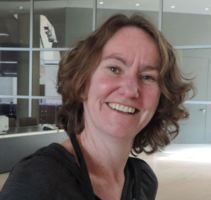
Research Directions:
- Develop deep neural networks based transfer learning and domain adaptation methods
- Develop genetic programming based methods for transfer learning and domain adaption, including both Genetic Programming-based methods for transfer learning and transfer learning methods for use in Genetic Programming
- Evolutionary computation, particularly Genetic Programming, based on evolving deep neural networks for transfer learning and domain adaption
Theme 4: Multi-criteria Optimisation and Decision Making
Leaders:
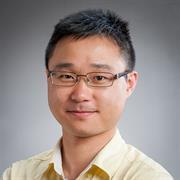
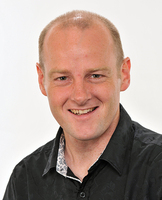
Research Directions:
- Doing research in multi-objective algorithm design for machine learning and hyper-heuristic approaches, such as Genetic Programming Hyper-Heuristic for multi-tasking and multi-objective job shop scheduling, and model performance vs complexity/size. We will continue these research directions and do more fundamental research (e.g. factoring multi-task learning, regularisation, non-stationarity and concept drift, and user-preferences in modelling)
- We will go ahead with the application-driven research, understand more of the breeding program design problem and collect the data, to see how to model the problem and design algorithms (or use existing algorithms) to solve it
Theme 5: Model Explicability and Visualisation
Leaders:
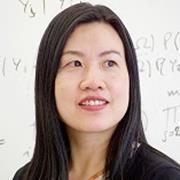
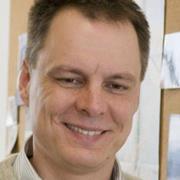
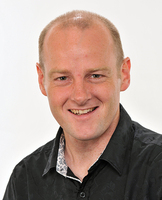
Research Directions:
- Methodology development using clustering for prediction (overlapped with finfish)
- Demonstration of the capabilities and limitations of causal learning in aquaculture-related domains, with an emphasis on capacity building and upskilling relevant stakeholders
- Develop new model complexity measures to improve the model explainability by reducing the model complexity
- Develop multi-objective methods to minimise both the error and model complexity
- Develop new visualisation methods